Modern Statistical Prediction and Machine Learning
Instruction Mode: In-Person Instruction
Open Seats
9 Unreserved Seats
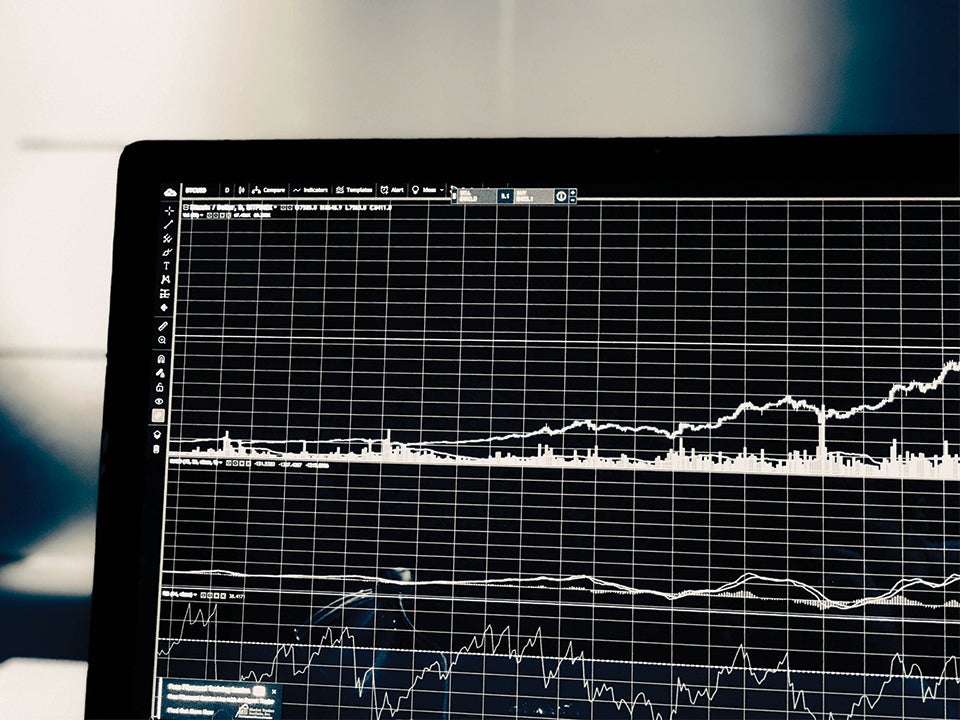
Instruction Mode: In-Person Instruction
Open Seats
9 Unreserved Seats
Instruction Mode: In-Person Instruction
Open Seats
13 Unreserved Seats
Instruction Mode: In-Person Instruction
Open Seats
22 Unreserved Seats
Instruction Mode: In-Person Instruction
Instruction Mode: In-Person Instruction
Instruction Mode: In-Person Instruction
Instruction Mode: In-Person Instruction
Instruction Mode: In-Person Instruction
Instruction Mode: In-Person Instruction
Instruction Mode: In-Person Instruction